The coronavirus pandemic has proved that the stock market is also volatile like all other business industries as it may crash within seconds and may also skyrocket in no time! Stocks are inexpensive at present because of this crisis and many people are involved in getting stock market data for helping with the informed options.
Unlike normal web scraping, extracting stock market data is much more particular and useful to people, who are interested in stock market investments.
Web Scraping Described
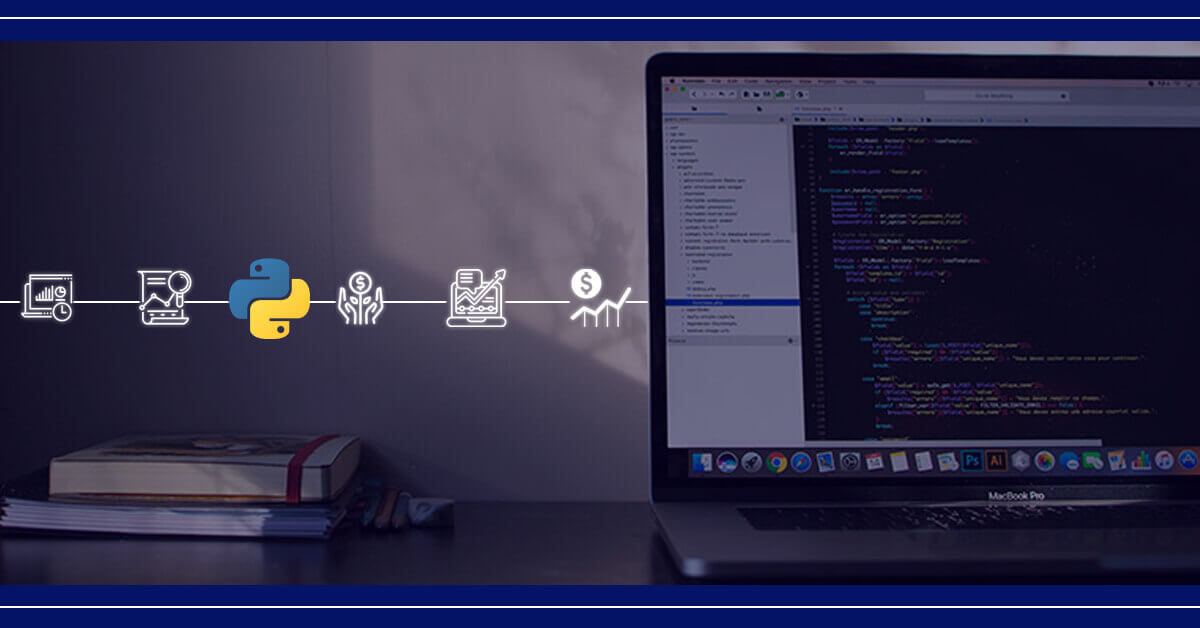
Web scraping includes scraping the maximum data possible from the preset indexes of the targeted websites and other resources. Companies use web scraping for making decisions and planning tactics as it provides viable and accurate data on the topics.
It's normal to know web scraping is mainly associated with marketing and commercial companies, however, they are not the only ones, which benefit from this procedure as everybody stands to benefit from extracting stock market information. Investors stand to take benefits as data advantages them in these ways:
- Investment Possibilities
- Pricing Changes
- Pricing Predictions
- Real-Time Data
- Stock Markets Trends
Using web scraping for others’ data, stock market data scraping isn’t the coolest job to do but yields important results if done correctly. Investors might be given insights on different parameters, which would be applicable for making the finest and coolest decisions.
Scraping Stock Market and Yahoo Finance Data with Python
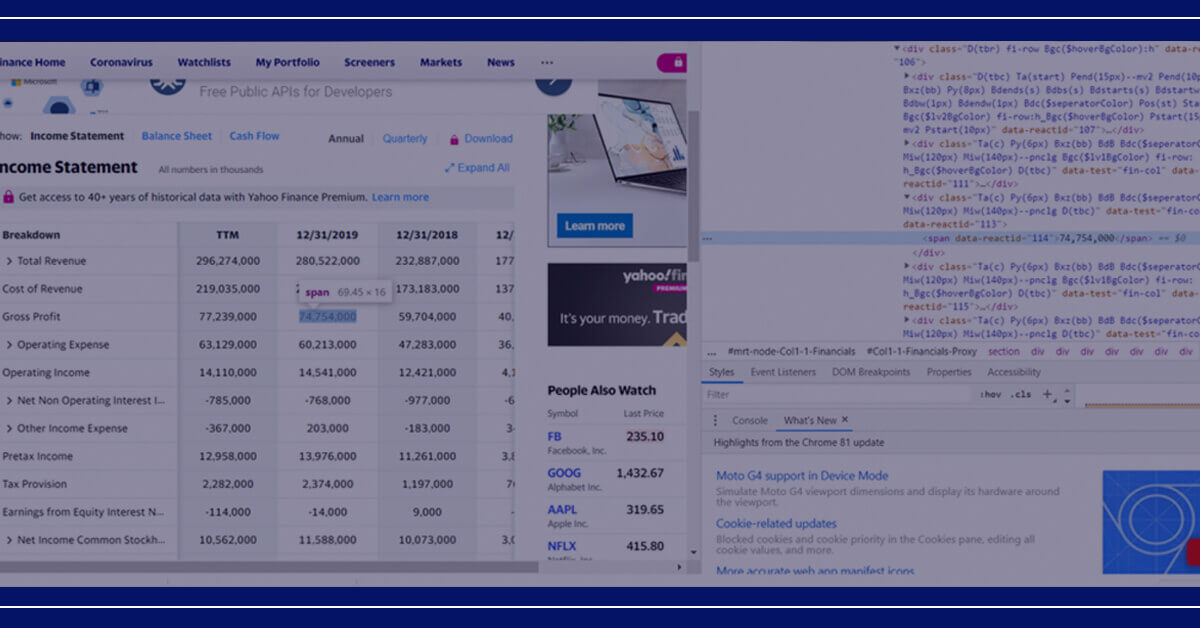
Initially, you’ll require installing Python 3 for Mac, Linux, and Windows. After that, install the given packages to allow downloading and parsing HTML data: and pip for the package installation, a Python request package to send requests and download HTML content of the targeted page as well as Python LXML for parsing with the Xpaths.
Python 3 Code for Scraping Data from Yahoo Finance
from lxml import html import requests import json import argparse from collections import OrderedDict def get_headers(): \ return {"accept": "text/html,application/xhtml+xml,application/xml;q=0.9,image/webp,image/apng,*/*;q=0.8,application/signed-exchange;v=b3;q=0.9", \ "accept-encoding": "gzip, deflate, br", \ "accept-language": "en-GB,en;q=0.9,en-US;q=0.8,ml;q=0.7", \ "cache-control": "max-age=0", \ "dnt": "1", \ "sec-fetch-dest": "document", \ "sec-fetch-mode": "navigate", \ "sec-fetch-site": "none", \ "sec-fetch-user": "?1", \ "upgrade-insecure-requests": "1", \ "user-agent": "Mozilla/5.0 (Windows NT 10.0; Win64; x64) AppleWebKit/537.36 (KHTML, like Gecko) Chrome/81.0.4044.122 Safari/537.36"} def parse(ticker): \ url = "http://finance.yahoo.com/quote/%s?p=%s" % (ticker, ticker) \ response = requests.get( \ url, verify=False, headers=get_headers(), timeout=30) \ print("Parsing %s" % (url)) \ parser = html.fromstring(response.text) \ summary_table = parser.xpath( \ '//div[contains(@data-test,"summary-table")]//tr') \ summary_data = OrderedDict() \ other_details_json_link = "https://query2.finance.yahoo.com/v10/finance/quoteSummary/{0}?formatted=true&lang=en-US®ion=US&modules=summaryProfile%2CfinancialData%2CrecommendationTrend%2CupgradeDowngradeHistory%2Cearnings%2CdefaultKeyStatistics%2CcalendarEvents&corsDomain=finance.yahoo.com".format( \ ticker) \ summary_json_response = requests.get(other_details_json_link) \ try: \ json_loaded_summary = json.loads(summary_json_response.text) \ summary = json_loaded_summary["quoteSummary"]["result"][0] \ y_Target_Est = summary["financialData"]["targetMeanPrice"]['raw'] \ earnings_list = summary["calendarEvents"]['earnings'] \ eps = summary["defaultKeyStatistics"]["trailingEps"]['raw'] \ datelist = [] \ for i in earnings_list['earningsDate']: \ datelist.append(i['fmt']) \ earnings_date = ' to '.join(datelist) \ for table_data in summary_table: \ raw_table_key = table_data.xpath( \ './/td[1]//text()') \ raw_table_value = table_data.xpath( \ './/td[2]//text()') \ table_key = ''.join(raw_table_key).strip() \ table_value = ''.join(raw_table_value).strip() \ summary_data.update({table_key: table_value}) \ summary_data.update({'1y Target Est': y_Target_Est, 'EPS (TTM)': eps, \ 'Earnings Date': earnings_date, 'ticker': ticker, \ 'url': url}) \ return summary_data \ except ValueError: \ print("Failed to parse json response") \ return {"error": "Failed to parse json response"} \ except: \ return {"error": "Unhandled Error"} if __name__ == "__main__": \ argparser = argparse.ArgumentParser() \ argparser.add_argument('ticker', help='') \ args = argparser.parse_args() \ ticker = args.ticker \ print("Fetching data for %s" % (ticker)) \ scraped_data = parse(ticker) \ print("Writing data to output file") \ with open('%s-summary.json' % (ticker), 'w') as fp: \ json.dump(scraped_data, fp, indent=4)
Real-Time Data Scraping
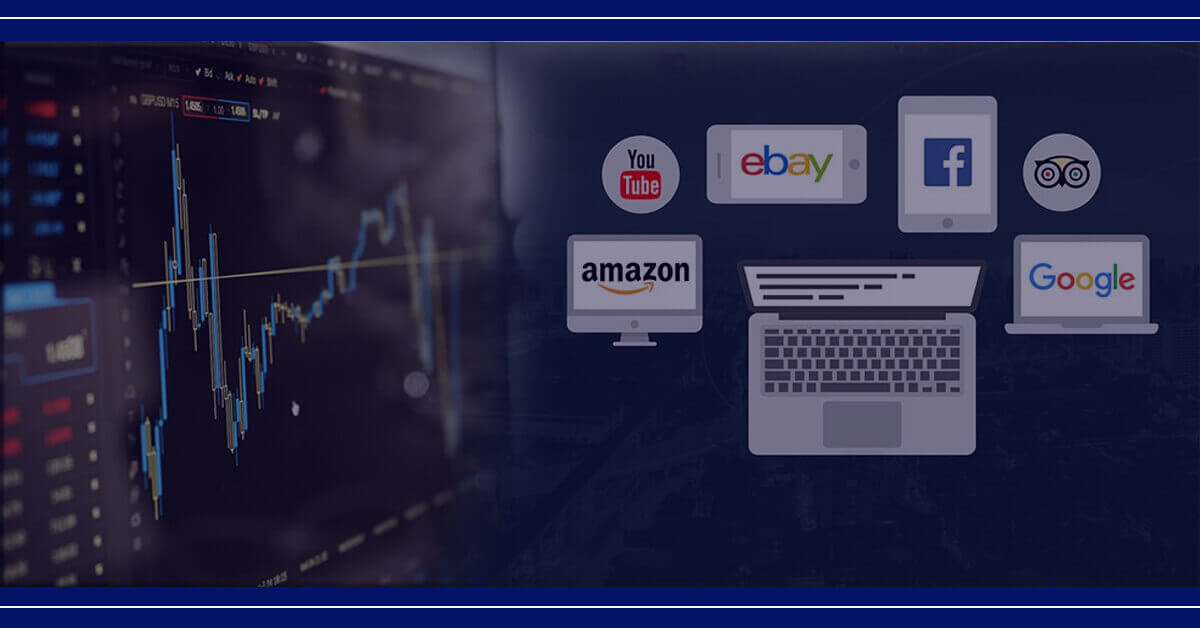
As the stock market has continuous ups and downs, the best option is to utilize a web scraper, which scrapes data in real-time. All the procedures of data scraping might be performed in real-time using a real-time data scraper so that whatever data you would get is viable then, permitting the best as well as most precise decisions to be done.
Real-time data scrapers are more costly than slower ones however are the finest options for businesses and investment firms, which rely on precise data in the market as impulsive as stocks.
Advantages of Stock Market Data Scraping

All the businesses can take advantage of web scraping in one form particularly for data like user data, economic trends, and the stock market. Before the investment companies go into investment in any particular stocks, they use data scraping tools as well as analyze the scraped data for guiding their decisions.
Investments in the stock market are not considered safe as it is extremely volatile and expected to change. All these volatile variables associated with stock investments play an important role in the values of stocks as well as stock investment is considered safe to the extent while all the volatile variables have been examined and studied.
To collect as maximum data as might be required, you require to do stock markets data scraping. It implies that maximum data might need to be collected from stock markets using stock market data scraping bots.
This software will initially collect the information, which is important for your cause as well as parses that to get studied as well as analyzed for smarter decision making.
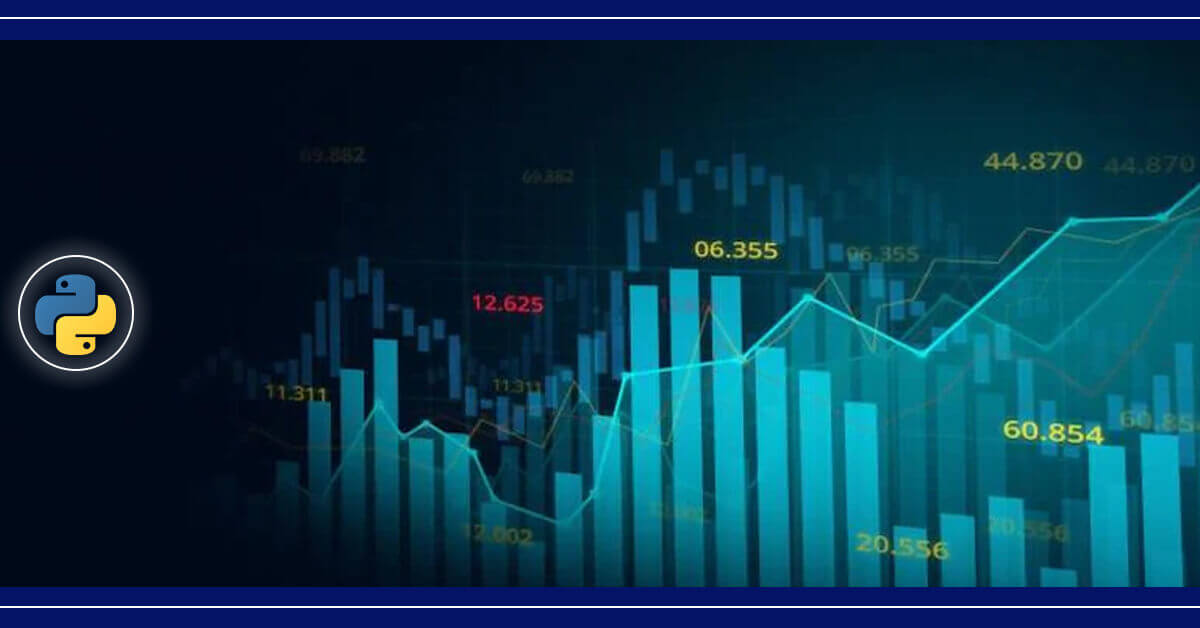
Jupyter notebook might be utilized in a course of the tutorial as well as you can have it on GitHub.
You will start installing jupyter notebooks because you have installed Anaconda
Along with anaconda, install different Python packages including beautifulsoup4, fastnumbers, and dill.
Add these imports to the Python 3 jupyter notebooks
import numpy as np # linear algebra import pandas as pd # pandas for dataframe based data processing and CSV file I/O import requests # for http requests from bs4 import BeautifulSoup # for html parsing and scraping import bs4 from fastnumbers import isfloat from fastnumbers import fast_float from multiprocessing.dummy import Pool as ThreadPool import matplotlib.pyplot as plt import seaborn as sns import json from tidylib import tidy_document # for tidying incorrect html sns.set_style('whitegrid') %matplotlib inline from IPython.core.interactiveshell import InteractiveShell InteractiveShell.ast_node_interactivity = "all"
What Will You Require Extract the Necessary Data?
Remove all the excessive spaces between the strings
A few strings from the web pages are available with different spaces between words. You may remove it with following:
def remove_multiple_spaces(string): \ if type(string)==str: \ return ' '.join(string.split()) \ return string
Conversions of Strings that Float
In different web pages, you may get symbols mixed together with numbers. You could either remove symbols before conversions, or you utiize the following functions:
def ffloat_list(string_list): \ return list(map(ffloat,string_list))
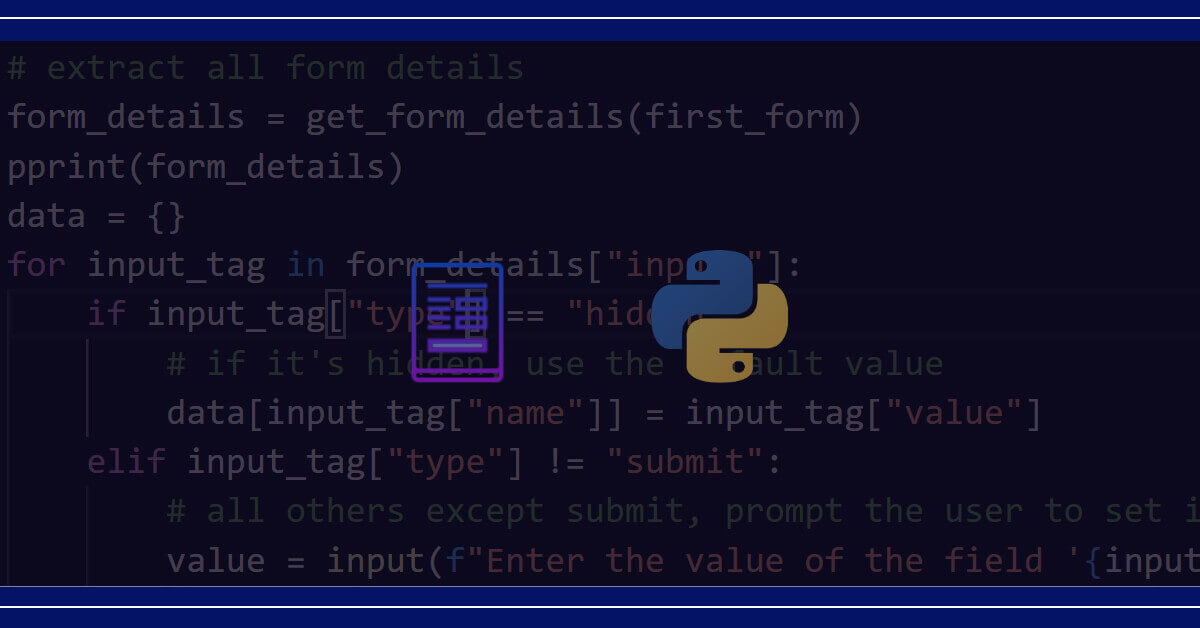
Before making any HTTP requests, you require to get a URL of a targeted website. Make requests using requests.get, utilize response.status_code for getting HTTP status, as well as utilize response.content for getting a page content.
Scrape and Parse the JSON Content from the Page
Scrape json content from the page with response.json() and double check using response.status_code.
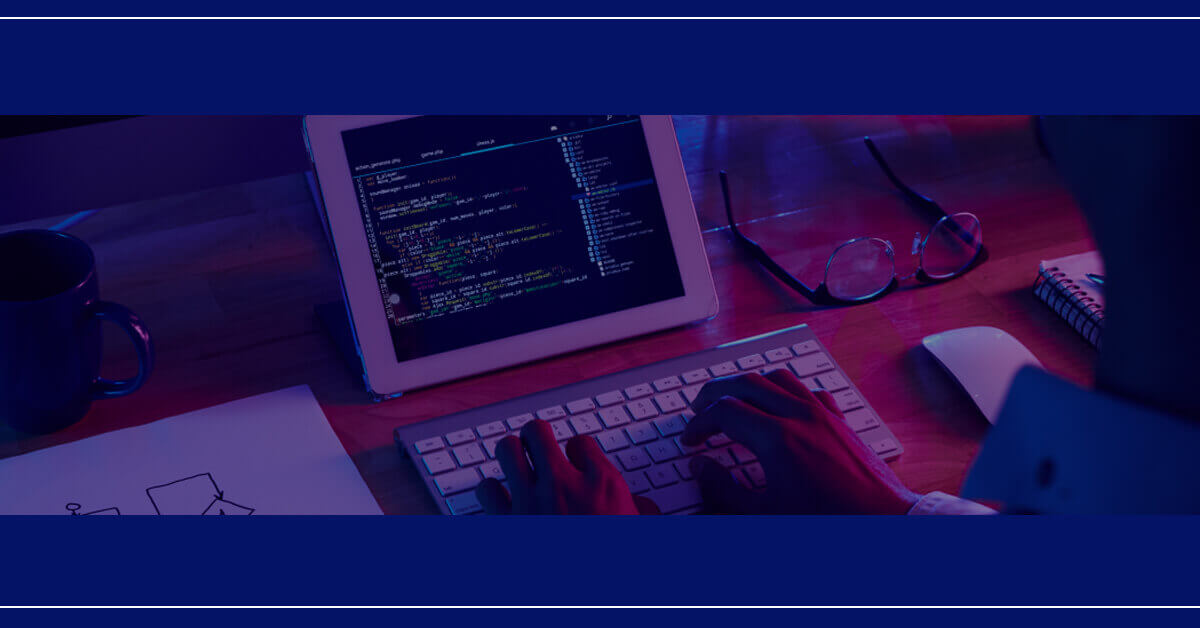
For that, we would use beautifulsoup4 parsing libraries.
Utilize Jupyter Notebook for rendering HTML Strings
Utilize the following functions:
from IPython.core.display import HTML HTML("Rendered HTML")
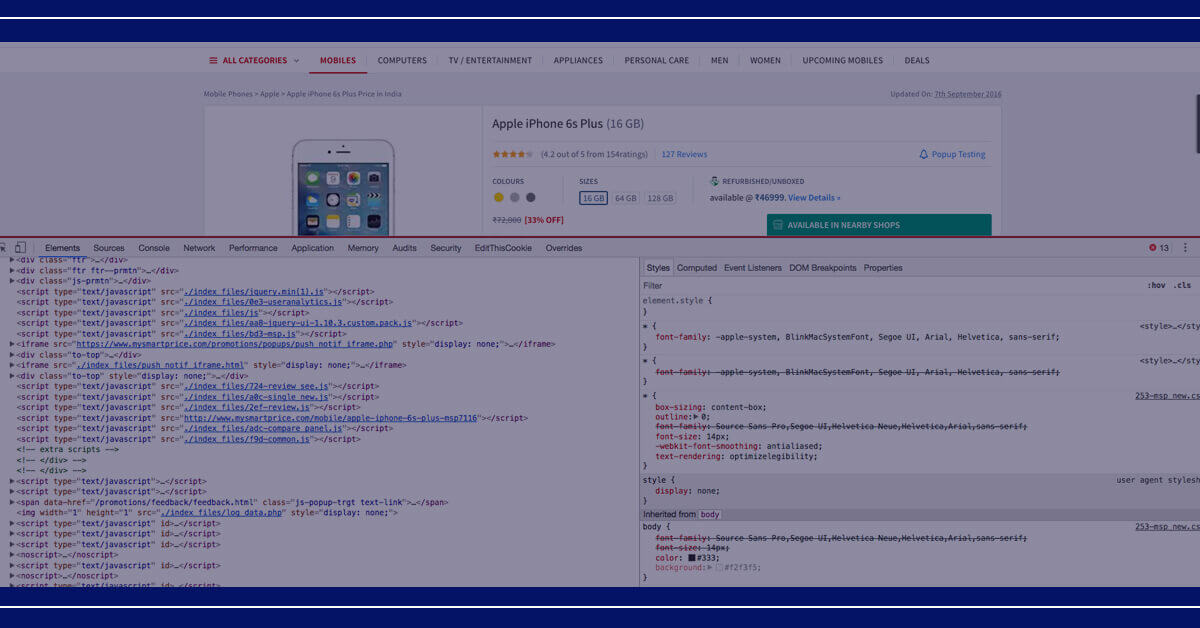
You’ll initially require to know HTML locations of content that you wish to scrape before proceeding. Inspect a page with Mac or chrome inspector using the functions cmd+option+I as well as inspect for the Linux using a function called Control+Shift+I.
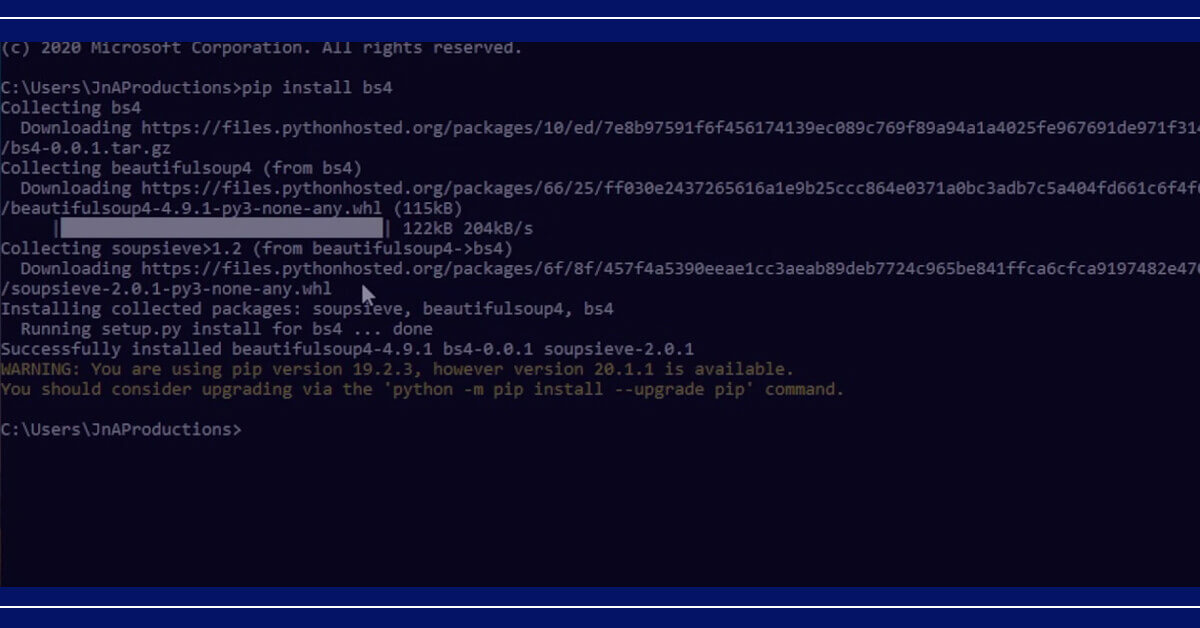
Parse a content with a function BeautifulSoup as well as get content from the header 1 tag as well as render it.
A web scraper tool is important for investment companies and businesses, which need to buy stocks as well as make investments in stock markets. That is because viable and accurate data is required to make the finest decisions as well as they could only be acquired by scraping and analyzing the stock markets data.
There are many limitations to extracting these data however, you would have more chances of success in case you utilize a particularly designed tool for the company. You would also have a superior chance in case, you use the finest IPs like dedicated IP addresses provided by Web Screen Scraping.
For more information, contact Web Screen Scraping!
Comments
Post a Comment