The stock market is a massive database for technological companies, with millions of records that are updated every second! Because there are so many companies that provide financial data, it's usually done through Real-time web scraping API, and APIs always have premium versions. Yahoo Finance is a dependable source of stock market information. It is a premium version because Yahoo also has an API. Instead, you can get free access to any company's stock information on the website.
Although it is extremely popular among stock traders, it has persisted in a market when many large competitors, including Google Finance, have failed. For those interested in following the stock market, Yahoo provides the most recent news on the stock market and firms.
Steps to Scrape Yahoo Finance
- Create the URL of the search result page from Yahoo Finance.
- Download the HTML of the search result page using Python requests.
- Scroll the page using LXML-LXML and let you navigate the HTML tree structure by using Xpaths. We have defined the Xpaths for the details we need for the code.
- Save the downloaded information to a JSON file.
We will extract the following data fields:
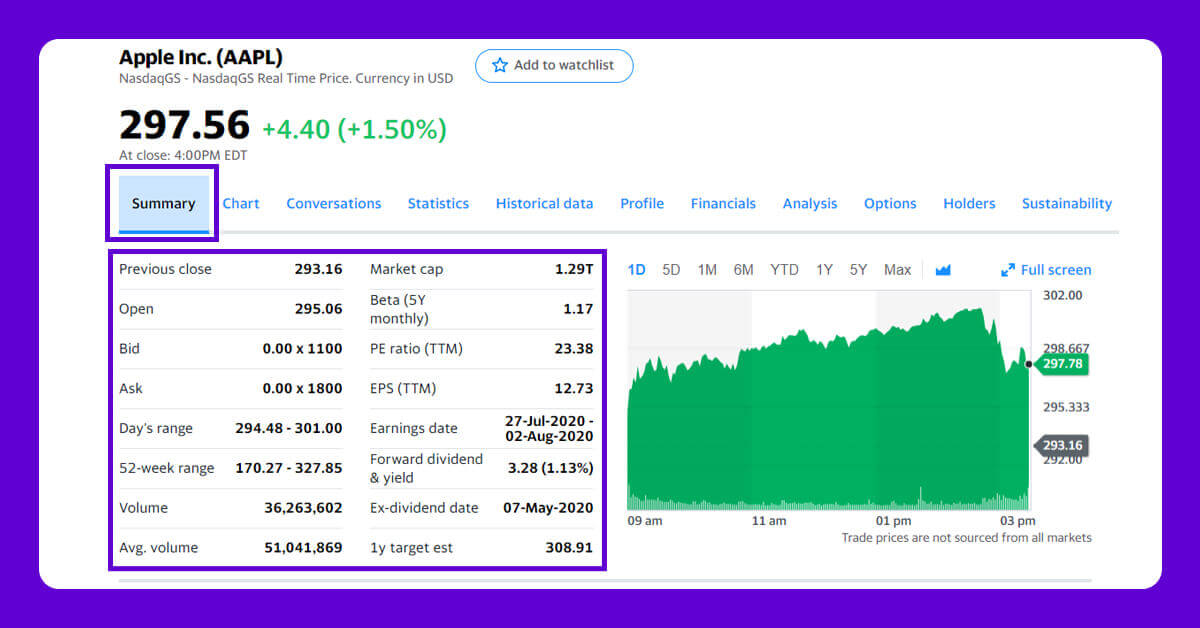
- Previous close
- Open
- Bid
- Ask
- Day’s Range
- 52 Week Range
- Volume
- Average volume
- Market cap
- Beta
- PE Ratio
- 1yr Target EST
You will need to install Python 3 packages for downloading and parsing the HTML file.
The Script
from lxml import html import requests import json import argparse from collections import OrderedDict def get_headers(): return {"accept": "text/html,application/xhtml+xml,application/xml;q=0.9,image/webp,image/apng,*/*;q=0.8,application/signed-exchange;v=b3;q=0.9", "accept-encoding": "gzip, deflate, br", "accept-language": "en-GB,en;q=0.9,en-US;q=0.8,ml;q=0.7", "cache-control": "max-age=0", "dnt": "1", "sec-fetch-dest": "document", "sec-fetch-mode": "navigate", "sec-fetch-site": "none", "sec-fetch-user": "?1", "upgrade-insecure-requests": "1", "user-agent": "Mozilla/5.0 (Windows NT 10.0; Win64; x64) AppleWebKit/537.36 (KHTML, like Gecko) Chrome/81.0.4044.122 Safari/537.36"} def parse(ticker): url = "http://finance.yahoo.com/quote/%s?p=%s" % (ticker, ticker) response = requests.get( url, verify=False, headers=get_headers(), timeout=30) print("Parsing %s" % (url)) parser = html.fromstring(response.text) summary_table = parser.xpath( '//div[contains(@data-test,"summary-table")]//tr') summary_data = OrderedDict() other_details_json_link = "https://query2.finance.yahoo.com/v10/finance/quoteSummary/{0}?formatted=true&lang=en-US®ion=US&modules=summaryProfile%2CfinancialData%2CrecommendationTrend%2CupgradeDowngradeHistory%2Cearnings%2CdefaultKeyStatistics%2CcalendarEvents&corsDomain=finance.yahoo.com".format( ticker) summary_json_response = requests.get(other_details_json_link) try: json_loaded_summary = json.loads(summary_json_response.text) summary = json_loaded_summary["quoteSummary"]["result"][0] y_Target_Est = summary["financialData"]["targetMeanPrice"]['raw'] earnings_list = summary["calendarEvents"]['earnings'] eps = summary["defaultKeyStatistics"]["trailingEps"]['raw'] datelist = [] for i in earnings_list['earningsDate']: datelist.append(i['fmt']) earnings_date = ' to '.join(datelist) for table_data in summary_table: raw_table_key = table_data.xpath( './/td[1]//text()') raw_table_value = table_data.xpath( './/td[2]//text()') table_key = ''.join(raw_table_key).strip() table_value = ''.join(raw_table_value).strip() summary_data.update({table_key: table_value}) summary_data.update({'1y Target Est': y_Target_Est, 'EPS (TTM)': eps, 'Earnings Date': earnings_date, 'ticker': ticker, 'url': url}) return summary_data except ValueError: print("Failed to parse json response") return {"error": "Failed to parse json response"} except: return {"error": "Unhandled Error"} if __name__ == "__main__": argparser = argparse.ArgumentParser() argparser.add_argument('ticker', help='') args = argparser.parse_args() ticker = args.ticker print("Fetching data for %s" % (ticker)) scraped_data = parse(ticker) print("Writing data to output file") with open('%s-summary.json' % (ticker), 'w') as fp: json.dump(scraped_data, fp, indent=4)
Executing the Scraper
Assuming the script is named yahoofinance.py. If you type in the code name in the command prompt or terminal with a -h.
python3 yahoofinance.py -h usage: yahoo_finance.py [-h] ticker positional arguments: ticker optional arguments: -h, --help show this help message and exit
The ticker symbol, often known as a stock symbol, is used to identify a corporation.
To find Apple Inc stock data, we would make the following argument:
python3 yahoofinance.py AAPL
This will produce a JSON file named AAPL-summary.json in the same folder as the script.
This is what the output file would look like:
{ "Previous Close": "293.16", "Open": "295.06", "Bid": "298.51 x 800", "Ask": "298.88 x 900", "Day's Range": "294.48 - 301.00", "52 Week Range": "170.27 - 327.85", "Volume": "36,263,602", "Avg. Volume": "50,925,925", "Market Cap": "1.29T", "Beta (5Y Monthly)": "1.17", "PE Ratio (TTM)": "23.38", "EPS (TTM)": 12.728, "Earnings Date": "2020-07-28 to 2020-08-03", "Forward Dividend & Yield": "3.28 (1.13%)", "Ex-Dividend Date": "May 08, 2020", "1y Target Est": 308.91, "ticker": "AAPL", "url": "http://finance.yahoo.com/quote/AAPL?p=AAPL" }
This code will work for fetching the stock market data of various companies. If you wish to scrape hundreds of pages frequently, there are various things you must be aware of.
Why Perform Yahoo Finance Data Scraping?
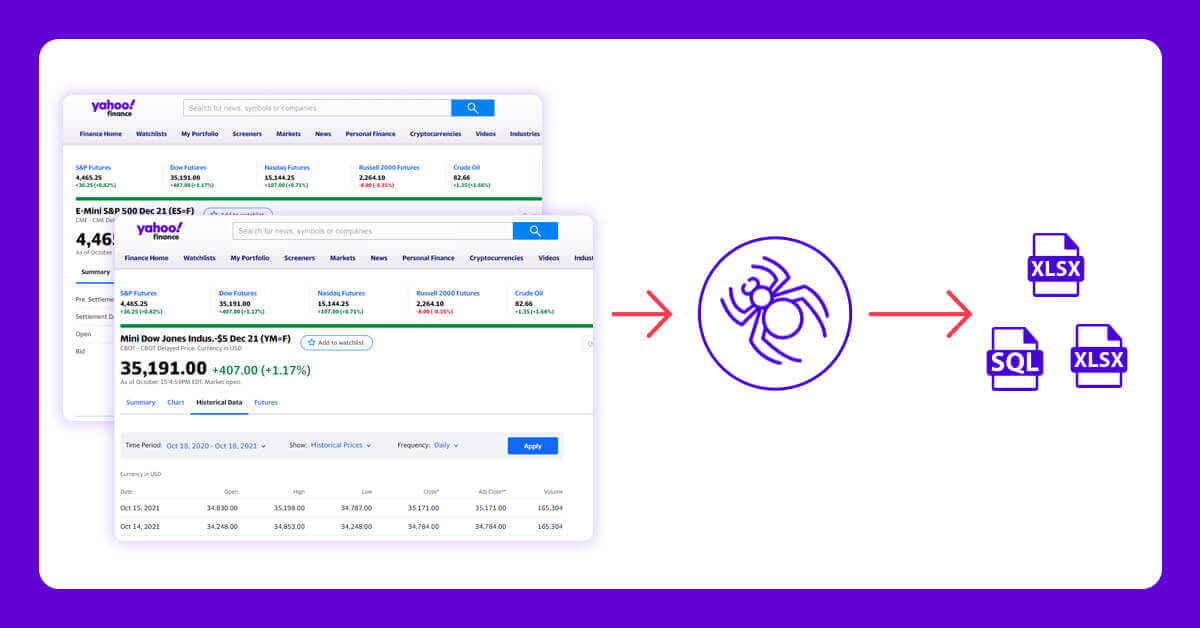
If you're working with stock market data and need a clean, free, and trustworthy resource, Yahoo Finance might be the best choice. Different company profile pages have the same format, thus if you construct a script to scrape data from a Microsoft financial page, you could use the same script to scrape data from an Apple financial page.
If anyone is unable to choose how to scrape Yahoo finance data then it is better to hire an experienced web scraping company like Web Screen Scraping.
For any queries, contact Web Screen Scraping today or Request for a free Quote!!
Comments
Post a Comment