This tutorial blog will tell you how to extract booking.com data for hotels with Selectorlib as well as Python. You may also use to scrape hotels data from Booking.com.
How to Extract Booking.com?
Search Booking.com for the Hotels data with conditions like Locations, Room Type, Check In-Check out Date, Total People, etc.
Copy the Search Result URL as well as pass that to the hotel scraper.
With the scraper, we would download the URL with Python Requests.
After that, we will parse the HTML with Selectorlib Template for scraping fields like Location, Name, Room Types, etc.
Then the scraper will save data into the CSV file.
The hotel scraper will scrape the following data. You can add additional fields also:
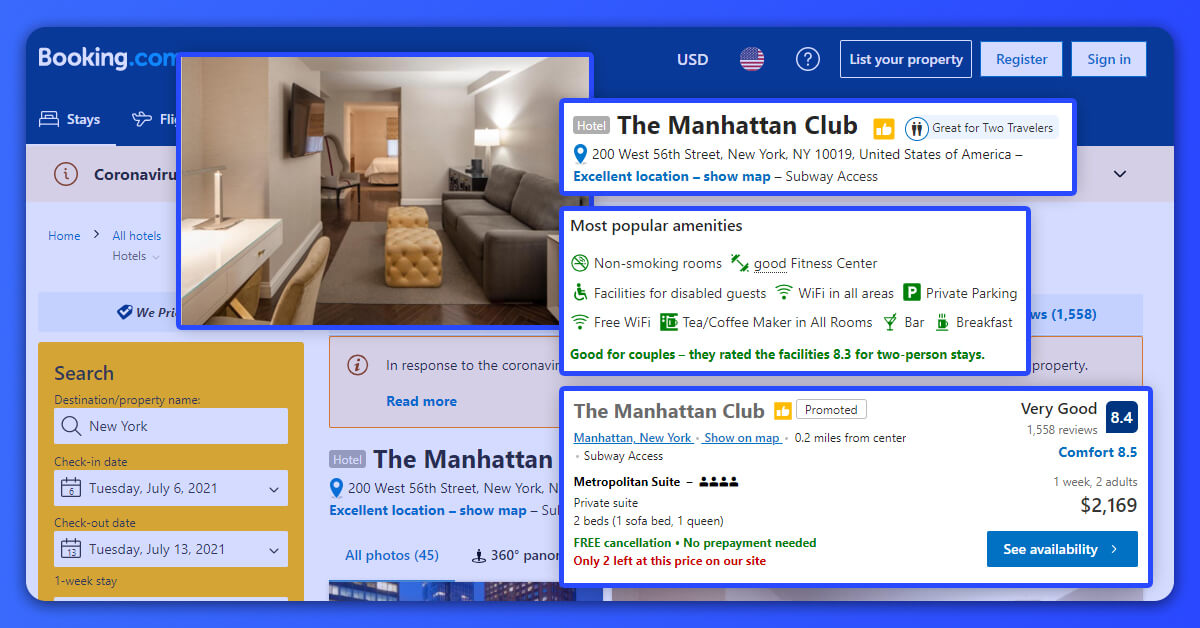
- Hotel’s Name
- Location
- Room Type
- Pricing
- Pricing For (eg: 2 Adults, 1 Night)
- Overall Ratings
- Bed Type
- Total Reviews
- Rating Tile
- Links
Installing the Packages Required to Run a Booking Data Scraper
We would require these Packages of Python 3
- Python Requests to do requests as well as downloading HTML content through Search Result pages from Booking.com. SelectorLib Python suites to extract data with YAML files that we have made from webpages, which we download.
Make installation using pip3
pip3 install requests selectorlib
The Code
It’s time to make a project folder named booking-hotel-scraper. In this folder, add one Python file named scrape.py
After that, paste the code given here in scrape.py
from selectorlib import Extractor import requests from time import sleep import csv # Create an Extractor by reading from the YAML file e = Extractor.from_yaml_file('booking.yml') def scrape(url): headers = { 'Connection': 'keep-alive', 'Pragma': 'no-cache', 'Cache-Control': 'no-cache', 'DNT': '1', 'Upgrade-Insecure-Requests': '1', # You may want to change the user agent if you get blocked 'User-Agent': 'Mozilla/5.0 (Macintosh; Intel Mac OS X 10_15_4) AppleWebKit/537.36 (KHTML, like Gecko) Chrome/81.0.4044.113 Safari/537.36', 'Accept': 'text/html,application/xhtml+xml,application/xml;q=0.9,image/webp,image/apng,*/*;q=0.8,application/signed-exchange;v=b3;q=0.9', 'Referer': 'https://www.booking.com/index.en-gb.html', 'Accept-Language': 'en-GB,en-US;q=0.9,en;q=0.8', } # Download the page using requests print("Downloading %s"%url) r = requests.get(url, headers=headers) # Pass the HTML of the page and create return e.extract(r.text,base_url=url) with open("urls.txt",'r') as urllist, open('data.csv','w') as outfile: fieldnames = [ "name", "location", "price", "price_for", "room_type", "beds", "rating", "rating_title", "number_of_ratings", "url" ] writer = csv.DictWriter(outfile, fieldnames=fieldnames,quoting=csv.QUOTE_ALL) writer.writeheader() for url in urllist.readlines(): data = scrape(url) if data: for h in data['hotels']: writer.writerow(h) # sleep(5)
This code will:
Open the file named urls.txt as well as download HTML content given for every link in that.
Parse this HTML with Selectorlib Template named booking.yml
Then save the output file in the CSV file named data.csv
It’s time to make a file called urls.txt as well as paste the search result URLs in it. Then we need to create a Selectorlib Template.
Make Selectorlib Template for Scraping Hotels Data from Booking.com Searching Results
You may notice that within a code given above, which we used the file named booking.yml. The file makes this code so short and easy. The magic after making this file is the Web Scraping tool called Selectorlib.
Selectorlib makes selecting, marking, as well as extracting data from the webpages visually easy. A Selectorlib Web Scraping Chrome Extension allows you to mark data, which you want to scrape, and makes CSS Selectors required for extracting the data. After that, preview how the data could look like.
In case, you require data that we have given above, you should not use Selectorlib. As we have already done it for you as well as produced an easy “template”, which you may use. Although, if you need to add new fields, you may use Selectorlib for adding those fields into a template.
Let’s see how we have moticed the data fields we needed to extract with Chrome Extension of Selectorlib.
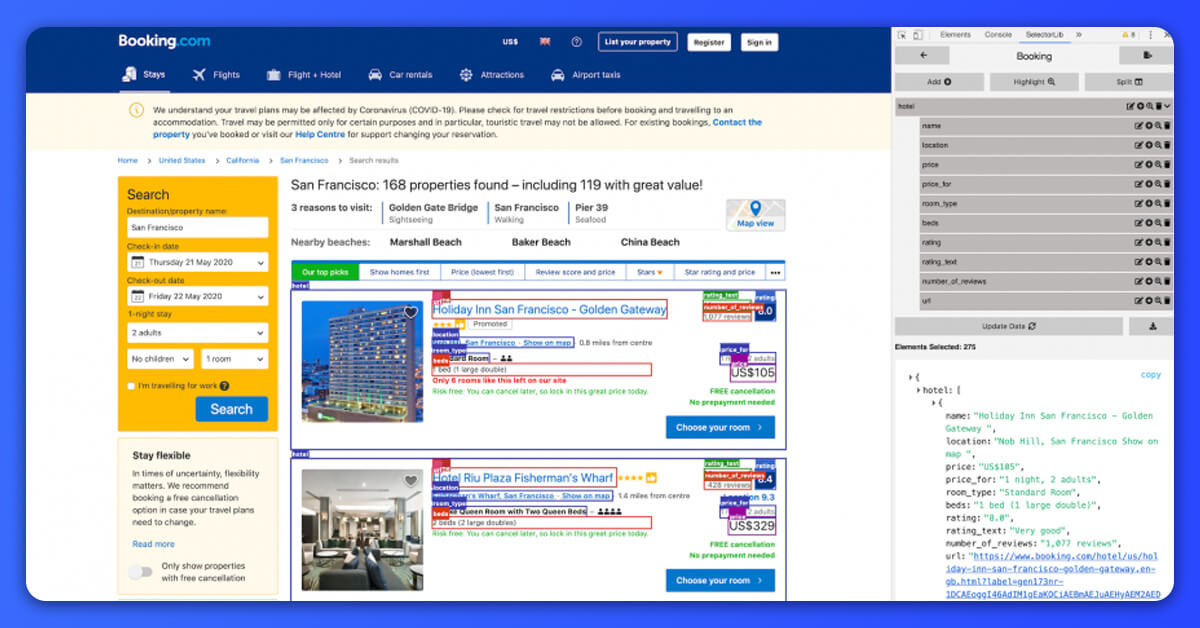
When you have made the template, just click on ‘Highlight’ button to highlight and preview all selectors. In the end, just click on ‘Export’ option and download YAML file, which is a booking.yml file.
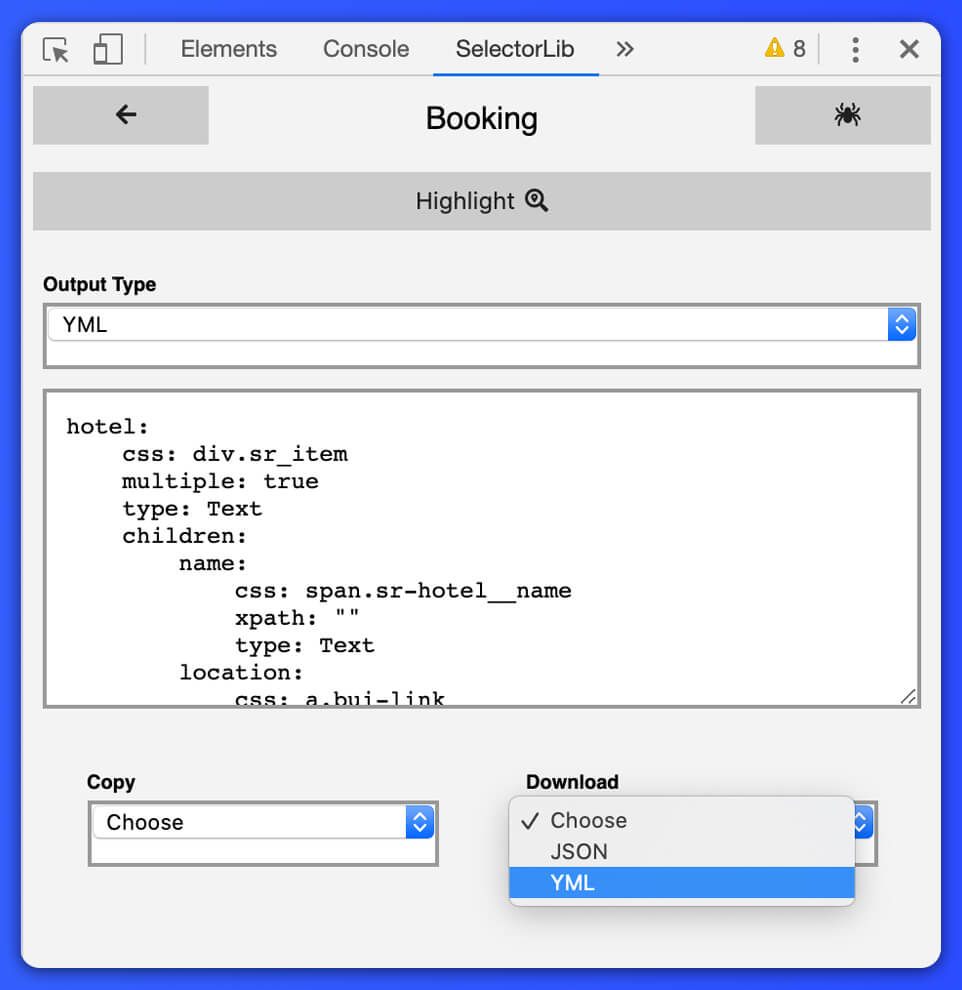
Let’s see how the template – booking.yml will look like:
hotels: css: div.sr_item multiple: true type: Text children: name: css: span.sr-hotel__name type: Text location: css: a.bui-link type: Text price: css: div.bui-price-display__value type: Text price_for: css: div.bui-price-display__label type: Text room_type: css: strong type: Text beds: css: div.c-beds-configuration type: Text rating: css: div.bui-review-score__badge type: Text rating_title: css: div.bui-review-score__title type: Text number_of_ratings: css: div.bui-review-score__text type: Text url: css: a.hotel_name_link type: Link
Run a Web Scraper
For running the web scraper, from a project folder,
- Try to search Booking.com to see your Hotels requirements
- Copy as well as add search results URLs into urls.txt
- Then Run the script python3 scrape.py
- Find data from the data.csv file
Let’s take a sample data from the search results pages.
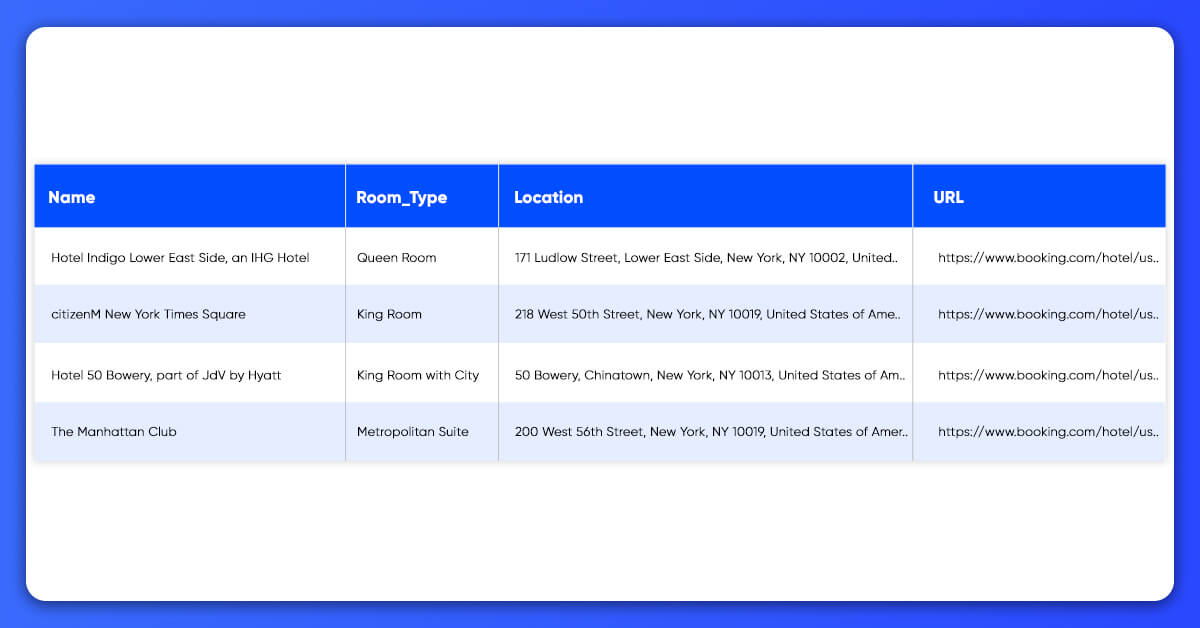
Contact Web Screen Scraping if you want to Extract Booking.com Data for Hotels!
Know More : https://www.webscreenscraping.com/how-to-extract-booking.com-data-for-hotels.php
Comments
Post a Comment